KI Workshop
intro-outro
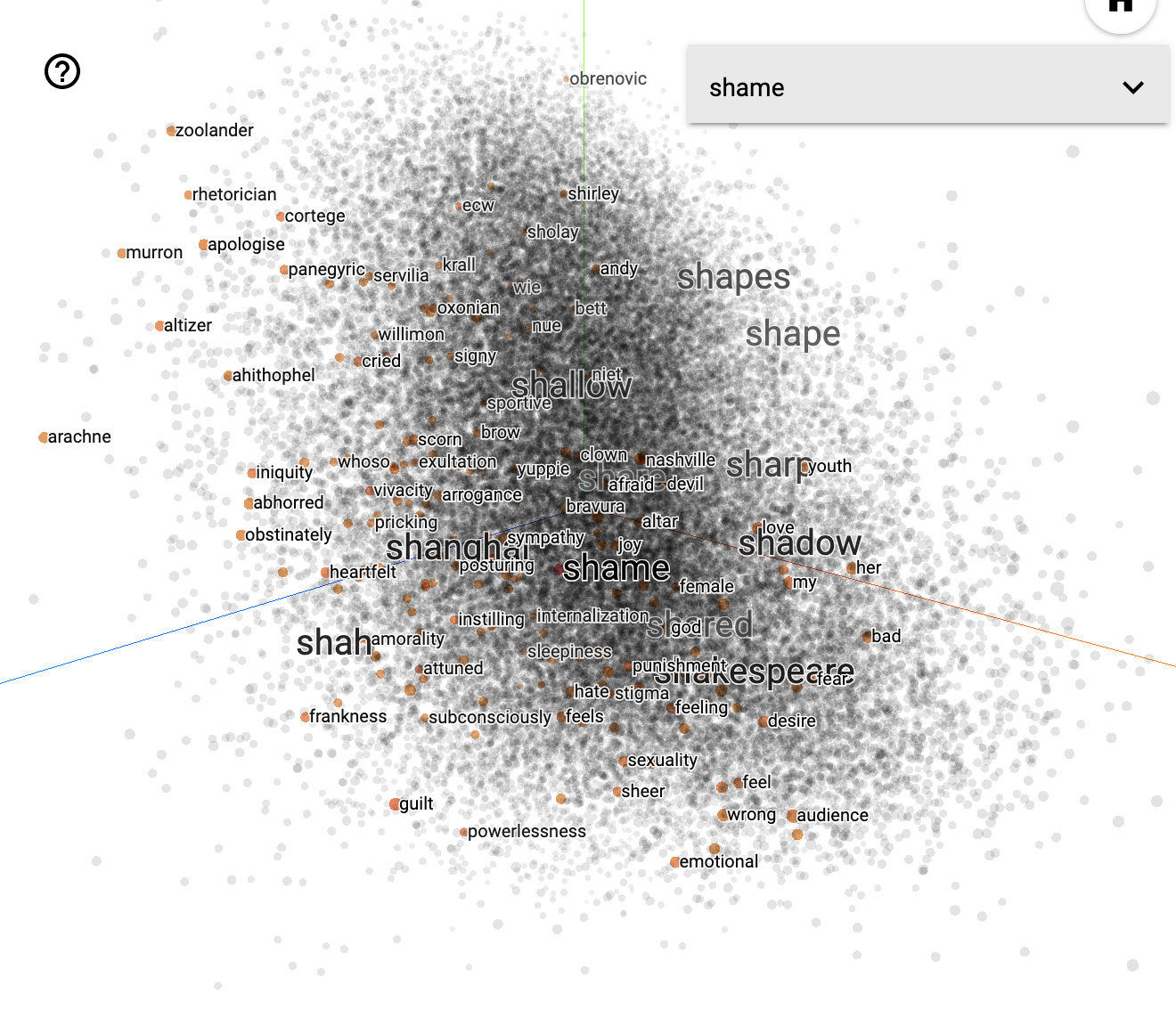
“It has been rightly urged that a history of brain models is really a history of the literary and material technologies which are familiar to, and then used as metaphors by, brain scientists. Their metaphorical menagerie exhibits mental clocks, logical pianos, barrel organisms, neural telegraphs and cerebral computer nets. How do specific technologies get into this zoo? Claims that certain systems can mimic, or even exhibit, intelligence are sustained by social hierarchies of head and hand. Minds are known because these social conventions are known. Simon Schaffer, ‘OK Computer’, 2001” (Pasquinelli, 2023, p. 133) (pdf)
“The wonder of our time, electrical telegraphy, was long ago modeled in the animal machine. But the similarity between the two apparatus, the nervous system and the electric telegraph, has a much deeper foundation. It is more than similarity; it is a kinship between the two, an agreement not merely of the effects, but also perhaps of the causes. Emil Du Bois-Reymond, On Animal Motion, 1851” (Pasquinelli, 2023, p. 133) (pdf)
- AI with very specific algos
- multidimensional statistical analysis
- pic of cloud of words as illustration of “space” and distances and correlation
- different genealogies of computation
- “intelligence” - from SciFi, Hollywood, silicon valley
- “military” - from Fridrich Kittler etc
- “division of labour” - computation is part of social institutes.
- Babbadge
- Alan Turing -> servants and masters
- metaphors - technical -> organic -> technical
- telegraph -> neurons in brain -> AI systems
- applied
- part of social institues
- fun / entertaiment / time killers / marketing / pr -> affective labour
- sorting / dividing people into categories / preemptive + politics
- communication
EXTRACTIVISM
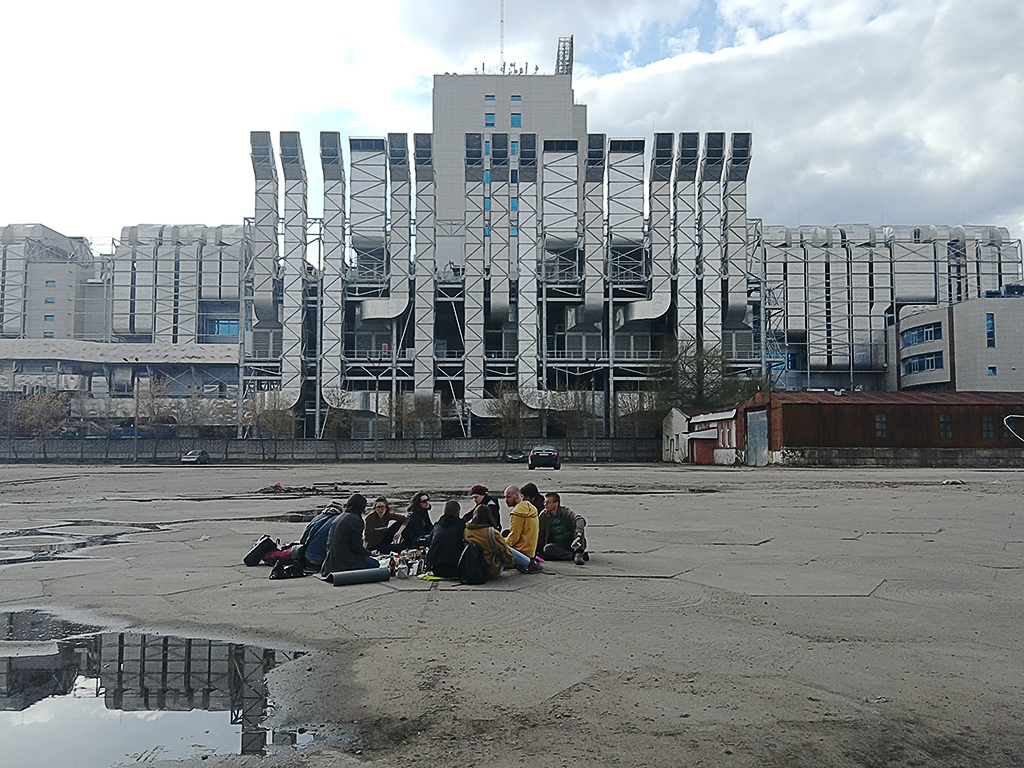
Discuss the New Extractivism video you have watched before this session.
The author Vladan Joler mentions that about some of these issues we don’t want to think about and simply ignoreOppressive A.I.: Feminist Categories to Understand its Political Effects
them, when generating AI images.
Do you agree? Discuss at the table.
Now read these quotes:
“The latest version of Stable Diffusion, an image generator, was trained on 256 A100 GPUs, or 32 machines with 8 A100s each, according to information online posted by Stability AI, totaling 200,000 compute hours. At the market price, training the model alone cost $600,000, Stability AI CEO Mostaque said on Twitter, suggesting in a tweet exchange the price was unusually inexpensive compared to rivals. That doesn’t count the cost of “inference,” or deploying the model.” (Leswing, 2023) (pdf)
““Now you could build something like a large language model, like a GPT, for something like $10, $20 million,” Huang said. “That’s really, really affordable.”” (Leswing, 2023) (pdf)
“environmental costs involved in these technologies, and OpenAI has not disclosed any specific data on infrastructure emissions.” (“Beyond the Faust and the Hype-Imaginaries of Large Language Text Models « INC Longform”) (pdf)
Find your position in this financial landscape. Share with others.
To make this landscape more material look through one of the map of datacentersData center is a physical building with shelves of hard drives, disks. Without them there can be no data, as it need an electricity, storage place and place to be processed. Please see the picture on top with a picnic near a data center.
or try to use something like google map.
- where are the most condensed areas? why?
- where is no data, or what places are not documented?
- how the appearance of such infrastructural object in one space can influence the urban tissue?
- where your data is stored? in which country, do you know?
Try to juxtapose the map proposed by Vladan Joler with the map of computation infrastructure.
INTIMATE INTERFACE
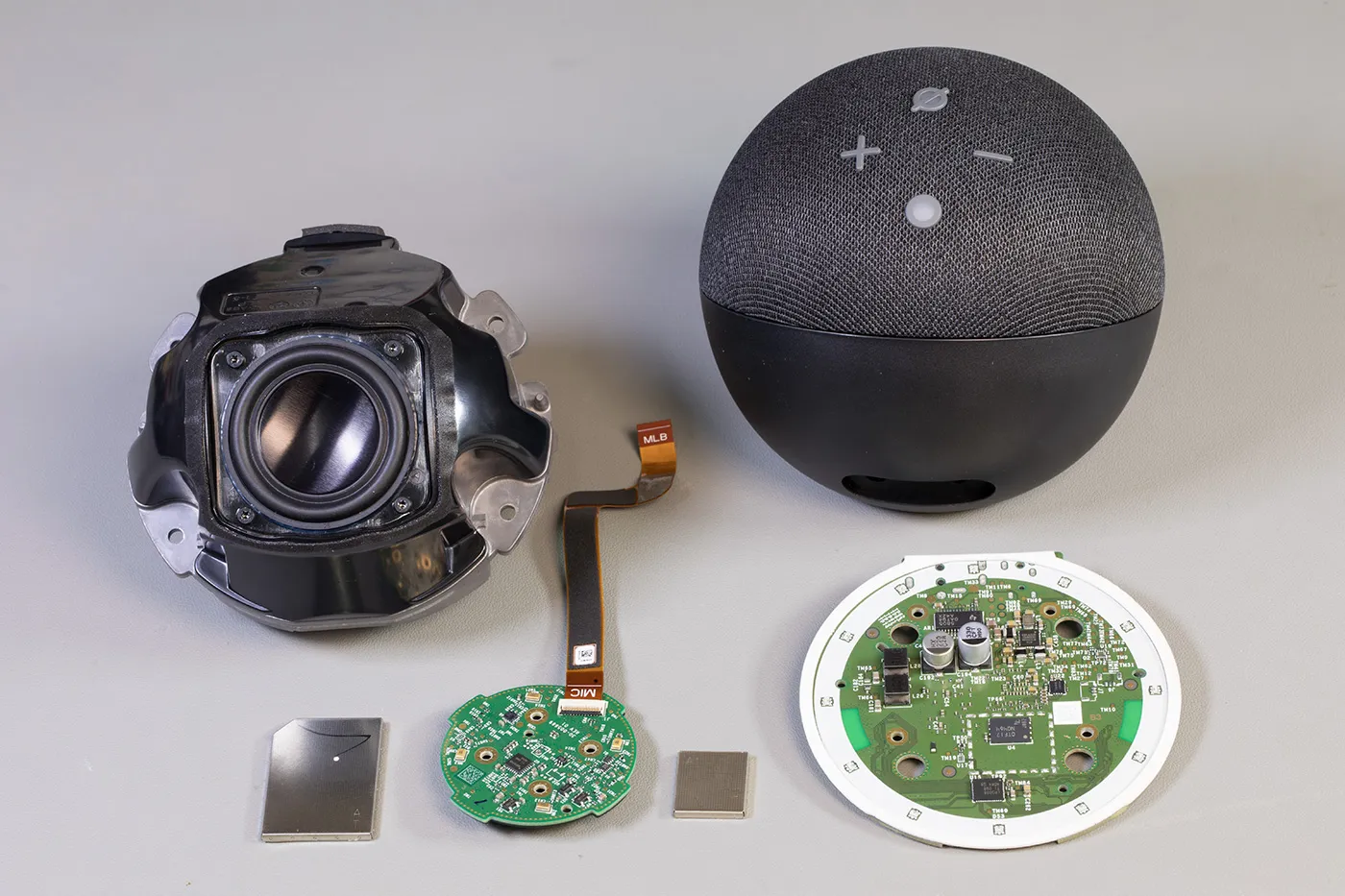
The exercise is to dive into the interface of automation of affect.
This instance that you see at the table was bought by us in Saturn two days ago. So it is the most actual version.
Disassemble the device till the moment when it will be important to talk about materials and parts it consists of. Use tools to dive into and navigate: screwdrivers. Use the map “anotomy of AI” to navigate through geographies and economies.
Questions to discuss in the group:
- what the package of the device could say us?
- what is the size of the device?
- what is the material?
- how does it smell?
- how big are different parts?
- is there any sign that indicates its origin from where it comes? did you look inside?
- Is it specially designed part or standard?
- what kind of metrics and measurements you could apply to it?
- do you have similar device at your home? wanna disassemble it too?
Draw a sort of report documenting the details, process and answers/discussion/critical moments. So people who will be after you at this table could read your observations.
In terms of urban life, do you find problematic the Amazon tower that is being built in Berlin now? Have you notice it? Have you been at the protests against it? What has been changed in Berlin since Amazon company came to the city - did you noticed some changes?
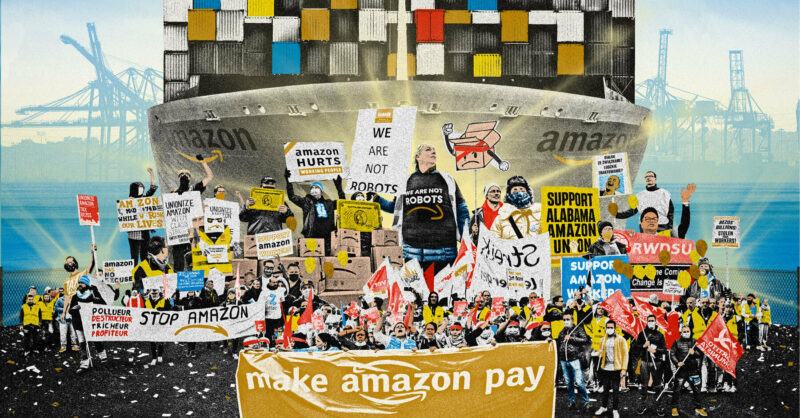
COMPAROLOGY
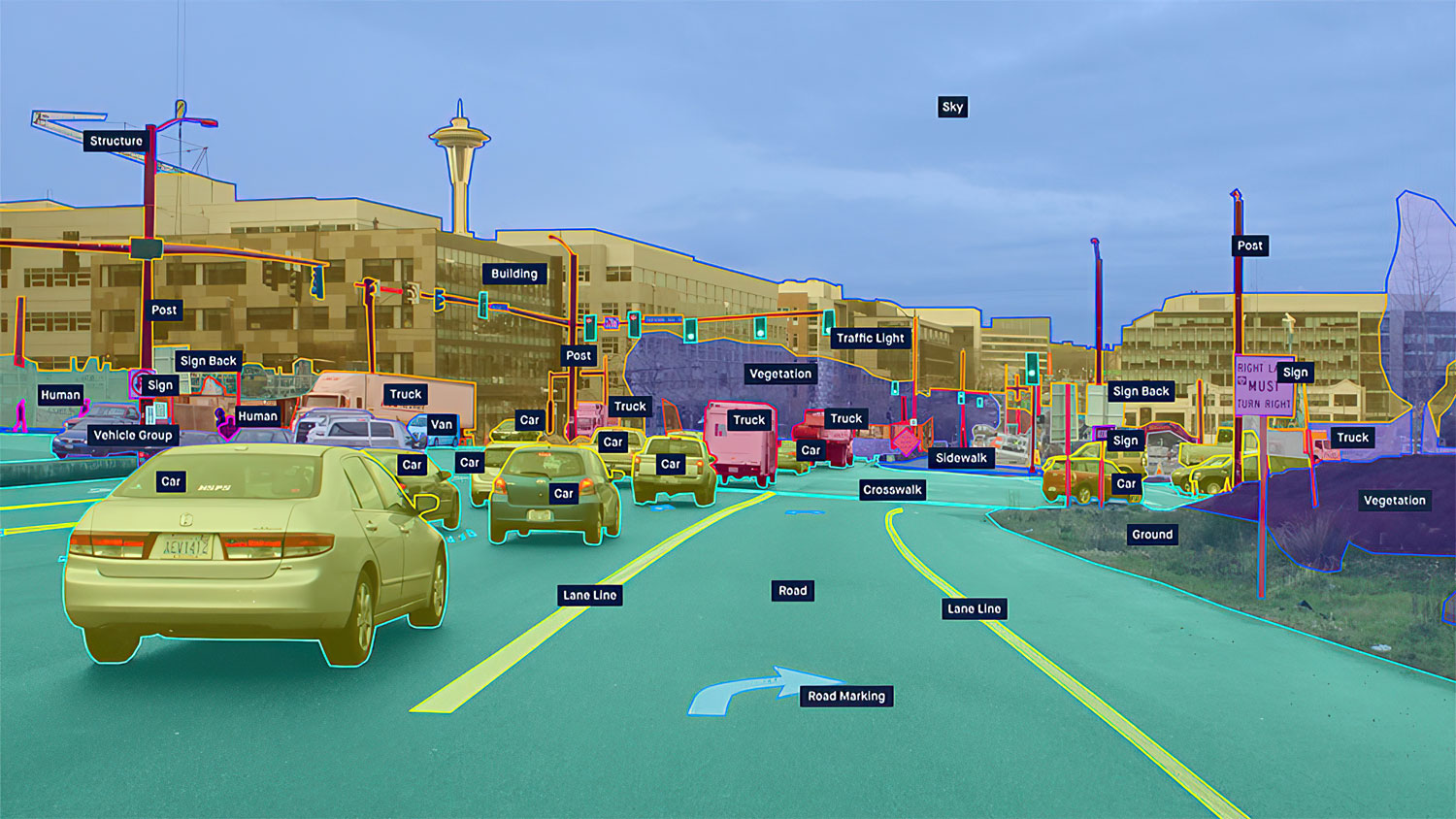
“I posted a HIT (Human Intelligence Tasks) on the Amacon Mechanical Turk* on March 19, 2015, titled Can you “tear” for me? and asked the online workers to upload pictures of themselves tearing for a stipend of 0.25D.” (“Xin Liu, artist and engineer, on earth and in outer space”) (pdf)
Let’s make an Exercise “Reverse engineeringReverse-engineering is the act of dismantling an object to see how it works. It is done primarily to analyze and gain knowledge about the way something works but often is used to duplicate or enhance the object.
of metrics”. What metricsif you need to understand what metrics could be, see these schemes: metrics connected to Affect or metrics connected to Impression
were used here? Please follow the link and enter your prompt:
To do this exercise use the same phrase in different image generating services. Try to find the phrase that can show the limits of the training data setDataset is a collection of structured data (pictures, texts, mathematical problems, weather data or in whatever domain you could think of) used to train statistical models (such as ChatGPT, stable diffusion etc). “datasets are a crucial component as they are core material used to train models.” (“Critical Dataset Studies Reading List”) (pdf)
. List of services:
- Stable Diffusion 2.1 – text-to-image model made by “Stability AI”
- DreamStudio - more extended version of this model
- Kandinsky 3.0 - Or try another image generatorListen to PR-people: “Training the ruDALL-E neural networks on the Christofari cluster has become the largest calculation task in Russia:” (“ruDALL-E: Generating Images from Text. Facing down the biggest computational challenge in Russia”, 2021) (pdf)
, made by of the Russian bank “Sberbank”. - Kandinsky 2.2
- Kandinsky 2.1
- Kandinsky
- Malevich
Discuss in the group the differences between generated pictures and what metrics were used to generate pictures: both inside of the interface
and hidden in statistical models. What was added? Changed? Censored? Banned? What is “beautiful”, what is not? Siblime? Antiaesthetics?
Discuss the parts of the interface. What controls (user interface elements) were used here? How owners of the services are trying to pull money out of you? Try different languages. How many words can you write?

what this labour interface could say about generated pictures that were given to us?
LABOUR INTERFACES
![Aaron Koblin - The Sheep Market[^sheep-market-info] Aaron Koblin - The Sheep Market“Processing drawing tool for recording sheep animations” (“Aaron Koblin - The Sheep Market”) (pdf)](images/draw-a-sheep.jpg)
Read about masters and servants:
“In a 1947 lecture, Alan Turing envisioned the Automatic Computing Engine (ACE), one of the first digital computers, as a centralised apparatus that orchestrated its operations as a hierarchy of master and servant roles:
Roughly speaking those who work in connection with the ACE will be divided into its masters and its servants. Its masters will plan out instruction tables for it, thinking up deeper and deeper ways of using it. Its servants will feed it with cards as it calls for them. They will put right any parts that go wrong. They will assemble data that it requires. In fact the servants will take the place of limbs. As time goes on the calculator itself will take over the functions both of masters and of servants. The servants will be replaced by mechanical and electrical limbs and sense organs. One might for instance provide curve followers to enable data to be taken direct from curves instead of having girls read off values and punch them on cards. The masters are liable to get replaced because as soon as any technique becomes at all stereotyped it becomes possible to devise a system of instruction tables which will enable the electronic computer to do it for itself. It may happen however that the masters will refuse to do this. They may be unwilling to let their jobs be stolen from them in this way. In that case they would surround the whole of their work with mystery and make excuses, couched in well chosen gibberish, whenever any dangerous suggestions were made. I think that a reaction of this kind is a very real danger.” (Pasquinelli, 2023, p. 7) (pdf)
Let’s play out division of labour gameOur mission is to organize mutual aid, resources, and advocacy to improve conditions for all people using Amazon’s Mechanical Turk (AMT) platform while striving to make this work a good job for all.
.
Part of your group pretends to be a “worker”, another part — a “manager”. Go to this website and make an account there according to your role in this game.
According to Slovenian artist Sanela Jahić, crowdworking can be described as a “global digital assembly line”More: OpenAI Used Kenyan Workers on Less Than $2 Per Hour to Make ChatGPT Less Toxic
, in which a single job is fragmented into many micro contracts and micropayments. (Bitnik et al., p. 24) (pdf)
Interface of worker
![ghostworker poem[^ghostworker-poem-info] ghostworker poemghostworkers poems (“Poems written by workers on Amazon Mechanical Turk. Anonymous crowdsourcing workers paid $0.05 for a poem.” (“Mechanical Turk Poems mturkpoems Instagram photos and videos”) (pdf))](images/turk-poem.png)
You see the interface of a micro task worker, or ghostworker, who label, sort, filter images and texts for AI models. First, choose a training or test task and make it together with a group of your worker-colleagues.
Second, see an interview and read the text and discuss the division of labour in these systems.
from Mean Images by Hito Steyerl:
“Creating filters to get rid of harmful and biased network outputs is a task increasingly outsourced to underprivileged actors, so-called microworkers, or ghostworkers. Microworkers identify and tag violent, biased and illegal material within datasets. They perform this duty in the form of underpaid ‘microtasks’ that turn digital pipelines into conveyor belts. As Time magazine reported in January 2023, underpaid workers in Kenya were asked to feed a network ‘with labeled examples of violence, hate speech and sexual abuse’.11 This detector is now used within Openai’s Chatgpt systems. In Western metropoles, microworkers are often recruited from constituencies that are barred from the official labour market by refugee or migrant legislation, as this anonymized interview with a digital worker in a large German city describes: Digital worker: We were all kind of in the same situation, in a very vulnerable situation. We were new to the city and to the country, trying to integrate, and we desperately needed a job. All the staff on my floor had at least a Master’s degree, I was not the only one. One of my colleagues was a biologist who specialized in butterfly research and had to work on the exact same tasks as I did. Because it was too difficult to find a real job, with a connection to her specialism, people just took this kind of part-time job. They were highly qualified people, with different language backgrounds. Interviewer: They were all foreigners? Digital worker: All of them. Interviewer: What was the work like? Digital worker: Terrible. And it was the same for everyone I met there. During training you are told that you’re going to see paedophilia, graphic content, sexually explicit language. And then when you actually start working, you sit at your desk and you see things which are unbelievable. Is this really true? The long-term effects of this work are pretty nasty. There was no one in my group who didn’t have problems afterwards. Problems like sleep disorders, loss of appetite, phobias, social phobia. Some even had to go to therapy. The first month you go through a very, very intense training. We had to learn how to recognize content that was too drastic. Because the ai or machine learning mechanisms were not able to decide the delicate cases. The machine has no feeling, it was not accurate enough. I became depressed. I had to go to therapy. I was prescribed medication. When I started there, my main job was to sieve through posts with sexually explicit content and so-called high priority cases, which usually had to do with suicide or self-harm. There were a lot of pictures of cutting. I had to analyse which were self-harm and which suicidal. The second month, I asked my team leader to put me in a different content workflow because I was feeling bad. Another interview conducted as part of the same project described how Syrian digital workers in Germany had to filter and review images of their own home towns, destroyed by the recent earthquake in the region—and, in some cases, the ruins of their former homes.13 They were deemed too violent for social media consumers, but not for the region’s inhabitants, who had been expelled by war and destruction and were forced to become ghostworkers in exile. Conveniently, military violence had provided digital corporations located in Germany with a new, supremely exploitable refugee workforce.” (Steyerl, 2023, p. 92) (pdf)
Interface of manager
You see the interface of a manager who needs a cheap labour to push forward their AI-ambissions. The manager who defines tasks, divides labour into micro pieces, bans workers for bad performance, rewards good ones. Try to be in their boots. Choose some of presets for tasks, look through demos and play with the interface.
Make it together with a group of your manager-colleagues.
Some of the templates (of tasks) you might find, include: - Survey Link - Survey - Image Classification - Bounding Box - Semantic Segmentation” - Instance Segmentation - Polygon - Keypoint - Image Contains - Video Classification - Moderation of an Image - Image Tagging - Image Summarization - Sentiment Analysis - Collect Utterance - Emotion Detection - Semantic Similarity - Conversation Relevance - Audio Transcription - Document Classification - Translation Quality - Audio Naturalness - Data Collection - Website Collection - Website Classification - Item Equality - Search Relevance” - (“Amazon Mechanical Turk - Requester UI Guide”, p. 5) (pdf)
- have you ever outsourced tasks to others?
- was that profitable? for you? for the worker?
- what kind of interfaces did you use?
DATASETS
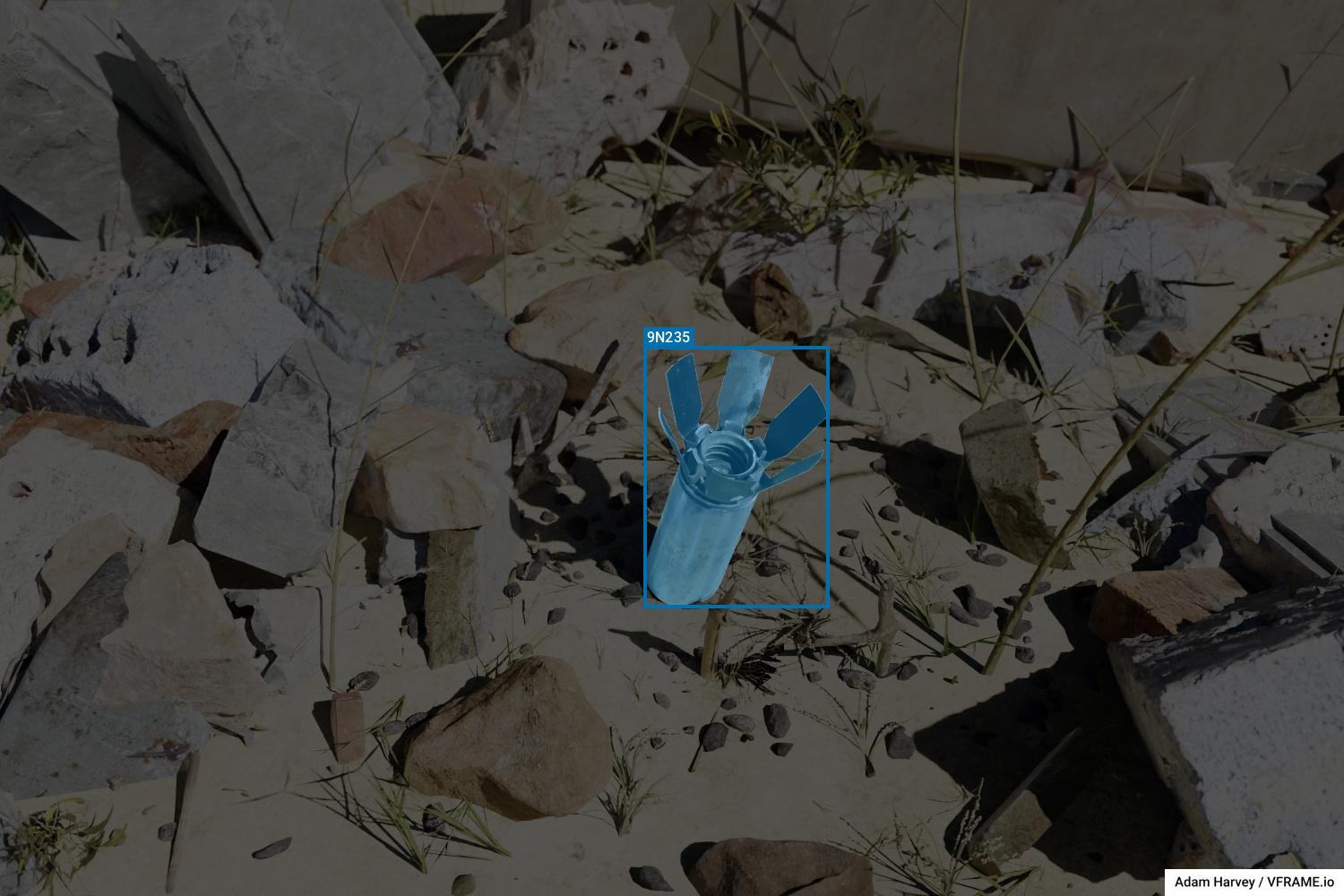
Remember that “data is a new oil”? one could say that who owns the data owns the world. do you agreeOften the tools I need to make Feminist Data Set as a critical design and art project don’t exist. / Feminist AI. Critical Perspectives on Algorithms, Data, and Intelligent Machines
? Another could say that privatising the data is the primary accumulation of capital of nowadays (primäre Kapitalakkumulation)
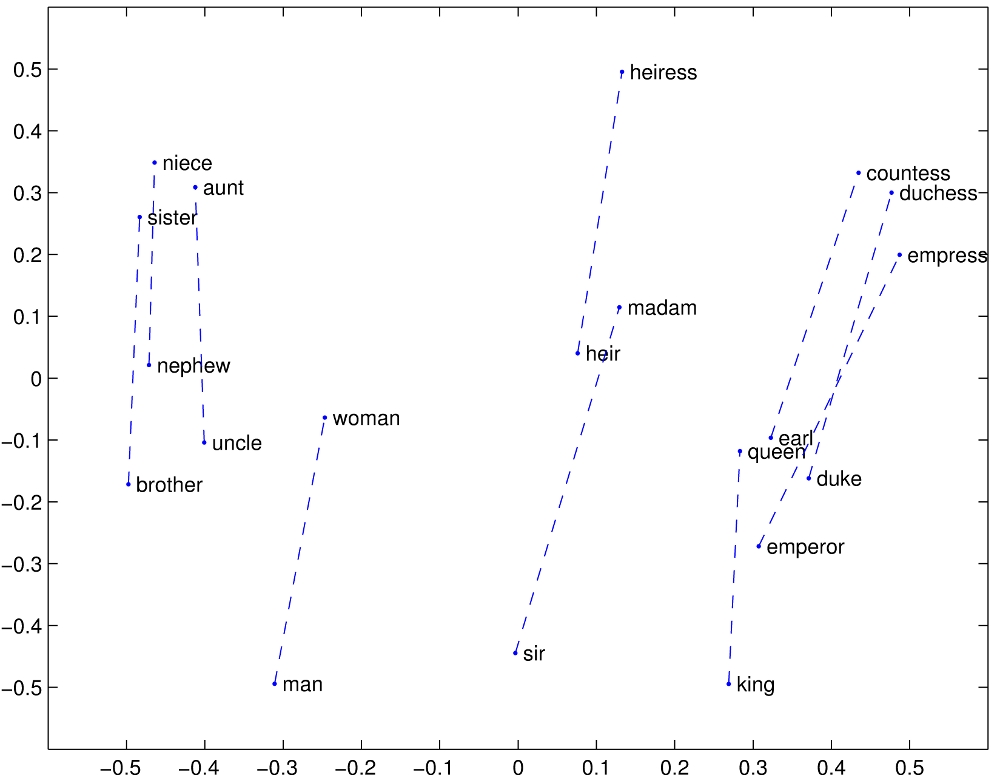
Below are some datasetsDataset is a collection of structured data (pictures, texts, mathematical problems, weather data or in whatever domain you could think of) used to train statistical models (such as ChatGPT, stable diffusion etc). “datasets are a crucial component as they are core material used to train models.” (“Critical Dataset Studies Reading List”) (pdf)
you can explore to unpack this. While going through them think about:
- what is quantified?
- what was calculated?
- what could not be calculated? if not so it is not a part of so called “AI”?
- who have made it? Was it paid work?
- who owned this data?
AVA: A Video Dataset of Spatio-temporally Localized Atomic Visual Actions
![Face recognition for chimpanzees. Photo: Kyoto University, Primate Research Institute[^ai-in-the-wild] Face recognition for chimpanzees. Photo: Kyoto University, Primate Research InstituteAI in the Wild. Sustainability in the Age of Artificial Intelligence](https://d2w9rnfcy7mm78.cloudfront.net/24817084/original_7cfb62956ae0f5c5de99a93391005bec.jpg?1700476565?bc=0)
The web interface for dataset you can find here
Answer questions in the group:
- what is sorted? how data is structured?
- what criteria are being used (on the left of interface)?
- what is the biggest category?
- what is the smallest?
- what is misrecognised?
- what does the company say?
ontological tree of words and concepts
First look into ontology of emotions made by user WNAffect. Now look through this tree find there the hierarchy of words of your choice. Here is better graphical interface
Here is another interface that could be used to explore mechanisms of formalisation of language.
Here you can see how different words and concepts relate to each other in a spacial model.
Search the words of your choice and discuss in the group the hierarchies of knowledge and power behind the dataset.
sea of datasets
Apart from language centric datasets there are sea of other data that is used to train predictionlike face recognition
or generativelike ChatGPT services
models of different spheres of human activities. For example, prediction of weather, “preemptive policing”“This Is a Story About Nerds and Cops”: PredPol and Algorithmic Policing by Jackie Wang
, “migration control”, recognition of “targets” during war conflicts “AI AT WAR. How Big Data, Artificial Intelligence, and Machine Learning Are Changing Naval Warfare” (“AI at war: how big data, artificial intelligence, and machine learning are changing naval warfare”, 2021, p. iii) (pdf)
, archeology, forensic journalismInvestigative series on Xinjiang detention camps
, medical insurance, sport bets.
What is quantified: Happy moments, Lottery Winning numbers, Beliefs, AI incidents., where Two planes too close, Humans in motion, Subnational conflicts. Look through the whole google-doc of descriptins and links to datasets or even a special data marketplace.
- what was the dynamics of datasets added to these services/docs?
- what is the difference in themes between years (2015-2023 for example)
- imagine sphere of human life and try to find quantified info there. decolonial practice? palestine?
LAION dataset
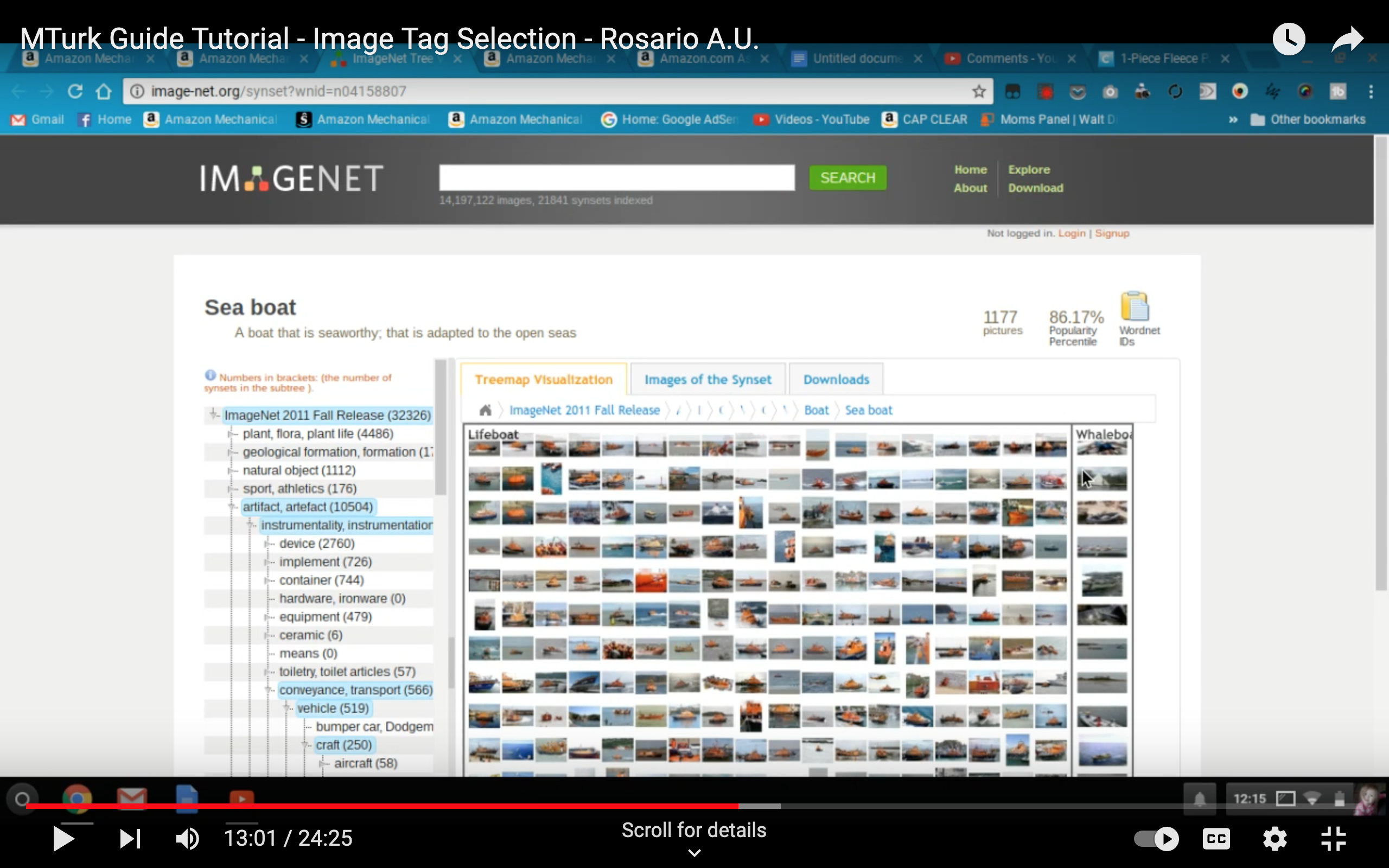
look through what was indexedLAION 5B is a large-scale dataset for research purposes consisting of 5,85Billions CLIP-filtered image-text pairs. 2,3Billions contain English language, 2,2Billions samples from 100+ other languages and 1Billions samples have texts that do not allow a certain language assignment (e.g. names ). Read what is behind this data
to make service like “stable diffusion” work.
- look through UI. What are the controls available to search through?
- try to play with
aesthetics
settings. What are the differences? - find some visuals related to your context. Compare results in the group .
- What is not in a dataset?
- can you find there a picture of youself?